Transformer Family on Time series
当代的时间序列分析任务需要应对各种复杂的数据模式和动态性,传统的统计模型和机器学习方法在处理这些挑战时常常受限。然而,近年来,类Transformer网络在时间序列分析领域取得了显著的突破。Transformer模型的出现为时间序列分析任务提供了一种新的、强大的工具,它能够自适应地捕捉序列中的长期依赖关系,并能够有效地建模非线性和非平稳性特征。
TODO: 完成重点论文和异常检测论文的精读
在文章中,我们将关注一些近年来在时间序列分析任务上涌现的重要工作,这些工作以类Transformer网络为基础,以其卓越的性能和创新的方法引起了广泛的关注。我们将重点介绍以下几个工作:
- N-BEATS(ICLR 2022)
- LogTrans(NeurIPS 2021)
- Informer ( AAAI 2021 Best Paper)📒Informer:用Transformer架构解决LSTF问题 - 知乎
- Autoformer(NeuraIPS 2021)📒细读好文 之 Autoformer - 知乎
- 🌟其中基于Wiener-Khinchin定理的Auto-Correlation Mechanism有点意思,可以单独看看。
- FEDformer(ICML 2022)📒阿里达摩院最新FEDformer,长程时序预测全面超越SOTA - 知乎
- Pyraformer(ICLR 2022)📒时间序列预测@Pyraformer - 知乎
- Transformer embeddings of irregularly spaced events and their participants(ICLR 2022)
- TranAD(VLDB 2022)
- Probabilistic Transformer For Time Series Analysis(NeurIPS 2021)。
🌟 此外,我们还将依托论文Are Transformers Effective for Time Series Forecasting?探讨一个重要的问题,即Transformer网络在时间序列预测中的有效性。
通过对这些工作的综述和分析,我们将深入了解类Transformer网络在时间序列分析任务中的应用,以及它们的创新之处、优点和局限性。这将有助于我们对该领域的最新研究进展有一个全面的了解,并为未来的研究和应用提供指导和启示。
Taxonomy of Transformers in Time Series
Yang, C., Mei, H., & Eisner, J. (2021). Transformer embeddings of irregularly spaced events and their participants. arXiv preprint arXiv:2201.00044.
根据上面的文章,基于Transformers的时间序列分析工作的创新点主要分为两类,即更改模型架构的,以及为特殊的应用而适配的。后文中,我们也将从这个分类法出发,来整理每个工作是如何改进Transformer的。
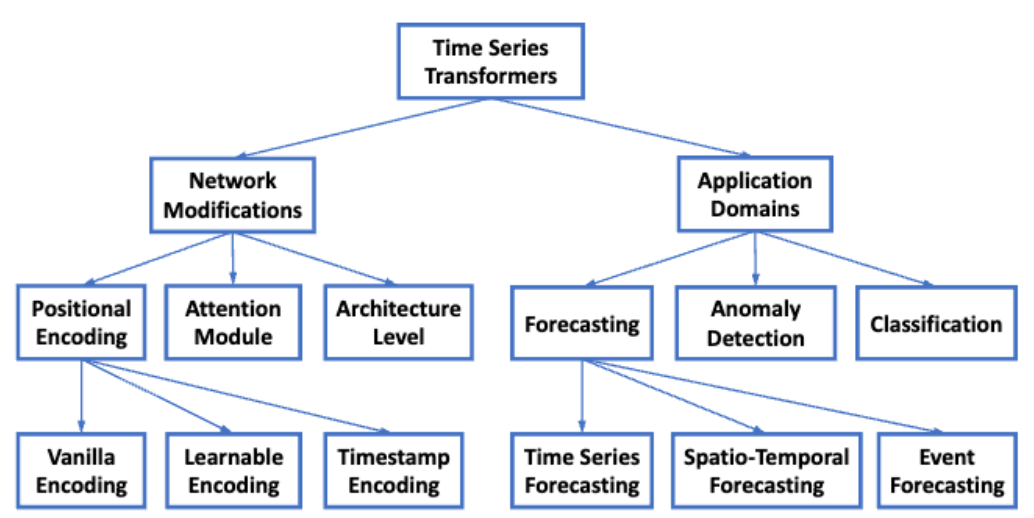
Network Modifications
Positional Encoding
- Learnable Positional Encoding
- Timestamp Encoding: Encoding calendar timestamps (e.g., second, minute, hour, week, month, and year) and special timestamps (e.g., holidays and events).
- Informer / Autoformer / FED former proposed to encode timestamps as additional positional encoding by using learnable embedding layers.
Attention Module
面向attention module的工作主要致力于减少self-attention module的时间、内存复杂度(原来为$\mathcal{O}(N^2)$)
- Introducing a sparsity bias into the attention mechanism: LogTrans, Pyraformer
- Exploring the low-rank property of the self-attention matrix to speed up the computation: Informer, FEDformer
Architecture-based Attention Innovation
这类工作直接面向时间序列的特殊性质,对Transformer的整体架构进行了改进
- Introduce hierarchical architecture into Transformer to take into account the multi-resolution aspect of time series: Informer, Pyraformer
- Informer: Inserts max-pooling layers with stride 2 between attention blocks, which down-sample series into its half slice (block-wise multi-resolution learning)
- Pyraformer: designs a C-ary tree-based attention mechanism, in which nodes at the finest scale correspond to the original time series, while nodes in the coarser scales represent series at lower resolutions.
- Pyraformer developed both intra-scale and inter-scale attentions in order to better capture temporal dependencies across different resolutions.
- Hierarchical architecture also enjoys the benefits of efficient computation, particularly for long-time series.
Application Domains
上面的综述对Forecasting、anomaly detection、Classification的相关研究都给出了详尽的调研,这里只整理与anomaly detection相关的内容。Transformer架构为anomaly detection任务做出的主要贡献还是“improve the ablity of modeling temporal dependency”。除此之外针对异常检测任务,常见的模型融合方式有:
- Combine Transformer with neural generative models: VAE – MT-RVAE, TransAnomaly; GAN – TranAD.
- Combine Transformer with graph-based learning architecture for multivariate time series anomaly detection: GTA
- Combine Transformer with Gaussian prior-Association: AnomalyTrans